PART 1 _ The Evolution and Future of AI in Market Research
- Cassiano Albuquerque
- Jun 25, 2024
- 4 min read
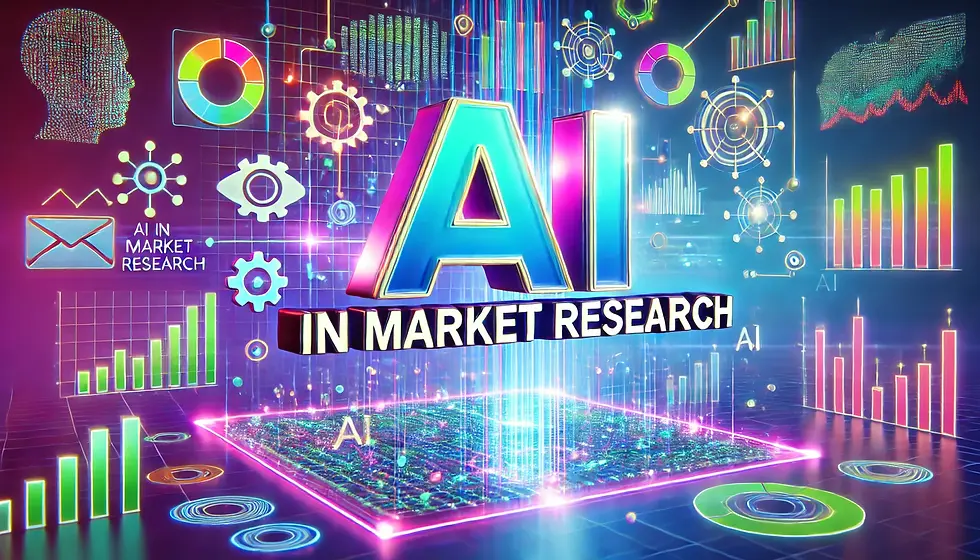
Artificial Intelligence (AI) is revolutionizing market research, offering unprecedented accuracy and depth in consumer insights. Reflecting on my journey in this field, I’ve witnessed AI's evolution firsthand, from early machine learning algorithms to today's sophisticated AI-driven analytics. This article explores the future of AI in market research, highlighting advancements in machine learning, integration with IoT and blockchain, AI-driven automation, personalization, data privacy, real-time analysis, and the essential balance between AI and human expertise.
Advancement of Machine Learning Algorithms in Market Research
Machine learning algorithms have evolved significantly, enabling researchers to analyze vast amounts of data with precision. These algorithms uncover hidden patterns and trends, enhancing market research quality. The development of deep learning has expanded these capabilities to analyze unstructured data like images, videos, and text, opening new avenues for understanding consumer behavior.
Real Cases:
1. Customer Sentiment Analysis
Coca-Cola has leveraged AI to dive deep into customer sentiment by analyzing over 120,000 pieces of customer feedback from social media. This analysis provided Coca-Cola with a granular understanding of consumer feelings about their brand, allowing them to adjust marketing strategies in real-time. By identifying common themes in the feedback, such as taste preferences and brand perception, Coca-Cola could refine its messaging and product offerings to better align with consumer expectations. This AI-driven approach not only enhanced customer satisfaction but also boosted engagement and loyalty (AI News).
2. Predictive Sales Models
Walmart employs advanced AI algorithms to forecast product demand with remarkable accuracy. By analyzing historical sales data, seasonal trends, and external factors like economic indicators, Walmart can predict future sales for various products. This predictive capability allows Walmart to optimize inventory levels, ensuring that popular items are always in stock while minimizing overstock of less popular products. The result is a significant reduction in waste and improved efficiency across their supply chain, ultimately leading to cost savings and better customer satisfaction (supply chain dive).
3. Product Recommendations
Amazon uses sophisticated AI algorithms to enhance the shopping experience through personalized product recommendations. By analyzing a customer's browsing history, previous purchases, and even items in their shopping cart, Amazon's AI can suggest products that are highly relevant to the individual. This personalized approach not only improves the customer experience but also significantly increases conversion rates and customer satisfaction. The recommendations engine is constantly learning and evolving, ensuring that suggestions remain relevant and timely (technologymagazine).
4. Visual and Audio Data Analysis
AI's ability to analyze visual and audio data provides deeper insights into consumer behavior that were previously unattainable. For instance, during focus group discussions, AI can evaluate facial expressions and vocal tones to gauge emotional reactions. This technology allows researchers to understand not just what participants are saying, but how they feel about the topics discussed. These insights are invaluable for creating more effective marketing strategies and product designs that resonate on an emotional level with consumers (Research World).
5. Churn Prediction
Telecom companies face a constant challenge in predicting customer churn. By analyzing usage patterns, customer service interactions, and social media activity, AI can identify customers at risk of leaving. This predictive insight allows telecom providers to proactively address customer concerns and improve service quality, thereby reducing churn rates. For example, by offering personalized retention strategies to at-risk customers, companies can increase loyalty and maintain a stable customer base (futurism technologies).
6. Dynamic Pricing Models
Retailers like Macy's use AI to implement dynamic pricing models that adjust prices in real-time based on demand and competitor pricing. This AI-driven approach allows Macy's to optimize revenue by increasing prices during high demand periods and offering discounts when demand is lower. The dynamic pricing strategy not only maximizes profit margins but also ensures that customers receive competitive prices, enhancing overall satisfaction and loyalty (BCG).
7. Ad Campaign Optimization
Google leverages AI to optimize ad campaigns by analyzing user interactions and adjusting bids in real-time. This continuous optimization ensures that ads are displayed to the most relevant audiences at the optimal times, maximizing the effectiveness of the campaigns. By using AI to monitor and adjust campaigns dynamically, Google helps advertisers achieve better results with lower costs and less manual intervention (spice works).
8. Customer Journey Mapping
Brands like Nike use AI to map customer journeys by analyzing interaction data across multiple touchpoints. This comprehensive view of the customer journey allows Nike to identify key moments that influence purchase decisions and optimize their marketing strategies accordingly. By understanding how customers move through the sales funnel, Nike can tailor its messaging and engagement efforts to better meet customer needs at each stage of the journey (QuestionPro).
9. Voice of the Customer Programs
Starbucks employs AI to analyze customer feedback from voice recordings and text responses. This analysis helps Starbucks identify common themes and issues that need addressing, allowing them to improve their service and products continuously. By listening to the voice of the customer in real-time, Starbucks can make swift adjustments to enhance customer satisfaction and loyalty (future stores).
10. Competitor Analysis
AI tools enable companies like Unilever to continuously monitor and analyze competitors' online activities and market strategies. This real-time competitive intelligence allows Unilever to stay ahead of industry trends and adjust its strategies proactively. By understanding competitors' strengths and weaknesses, Unilever can identify opportunities for differentiation and innovation, ensuring they remain a market leader (Unilever).
Conclusion
The advancement of machine learning algorithms is profoundly transforming market research. By enabling deeper insights and more accurate predictions, AI is helping businesses understand their customers better and make more informed decisions. As AI continues to evolve, its integration into market research will only become more sophisticated, offering even greater potential for innovation and efficiency.
By diving into these advancements and real-world applications, we can better appreciate the transformative power of AI in market research and anticipate the exciting developments yet to come.
Comments